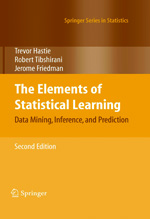
- The elements of statistical learning : data mining, inference, and prediction : 2nd ed.
- Tác giả: Trevor Hastie, Robert Tibshirani, J H Friedman
- Nhà xuất bản: Springer - New York
- Năm xuất bản: 2017
- Số trang:745 p.
- Kích thước:25 cm
- Số đăng ký cá biệt:44548
- ISBN:978-0387848570
- Mã Dewey:519.5
- Đơn giá:0
- Vị trí lưu trữ:03 Quang Trung
- Ngôn ngữ:English
- Loại tài liệu:Sách Chuyên ngành
- Đang rỗi/ Tổng sách:9/10
- Từ khóa:Supervised learning (Machine learning), Electronic data processing, Statistics
- Chủ đề: Statistics & Electronic data processing & Supervised learning (Machine learning)
- Chuyên ngành: Khoa Công Nghệ Thông Tin
- Tóm tắt: Contents: 1. Introduction; 2. Overview of supervised learning; 3. Linear methods for regression; 4. Linear methods for classification; 5. Basis expansions and regularization; 6. Kernel smoothing methods; 7. Model assessment and selection; 8. Model inference and averaging; 9. Additive models, trees, and related methods; 10. Boosting and additive trees; 11. Neural networks; 12. Support vector machines and flexible discriminants; 13. Prototype methods and nearest-neighbors; 14. Unsupervised learning; 15. Random forests; 16. Ensemble learning; 17. Undirected graphical models; 18. High-dimensional problem.
- Sách điện tử:http://thuvienso.duytan.edu.vn/handle/123456789/62867
Sách cùng chuyên ngành
Sách cùng chủ đề
- Time series analysis and its applications : with R examples : 4th ed.
- Big data analytics : from strategic planning to enterprise integration with tools, techniques, NoSQL, and graph
- Computational thinking
- Understanding distributed systems : what every developer should know about large distributed applications
- Data management at Scale : best practices for enterprise architecture